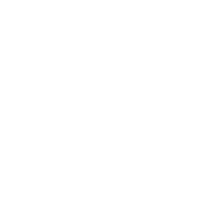
Song Han - TinyML: Reducing the Carbon Footprint of Artificial Intelligence in the Internet of Things (IoT)
Deep learning is computation-hungry and data-hungry. We aim to improve the computation efficiency and data efficiency of deep learning. I will first talk about MCUNet[1] that brings deep learning to IoT devices. The technique is tiny neural architecture search (TinyNAS) co-designed with a tiny inference engine (TinyEngine), enabling ImageNet-scale inference on an IoT device with only 1MB of FLASH. Next I will talk about TinyTL[2] that enables on-device training, reducing the memory footprint by 7-13x. Finally, I will describe Differentiable Augmentation[3] that enables data-efficient GAN training, generating photo-realistic images using only 100 images, which used to require tens of thousand of images. We hope such TinyML techniques can make AI greener, faster, and more sustainable.
Więcej odcinków z kanału "Stanford MLSys Seminar"
Nie przegap odcinka z kanału “Stanford MLSys Seminar”! Subskrybuj bezpłatnie w aplikacji GetPodcast.