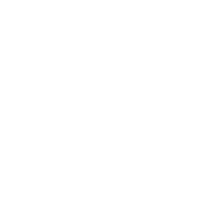
0:00
57:10
My guest for Episode 103 is Ronald Richman, FIA, FASSA, FeASK, CPCU, insurtech founder and thought leader in the actuarial data science movement.
The theme for the episode is 𝗗𝗲𝗲𝗽 𝗟𝗲𝗮𝗿𝗻𝗶𝗻𝗴.
Ron and I covered the following topics:
✅ Statistics, machine learning, deep learning, and generative AI
✅ Whether GLMs are statistical or machine learning models
✅ Neural nets and their applicability to insurance
✅ Reducing the risk of overparameterization for neural nets
✅ Balancing accuracy, complexity, and explainability for deep learning models
✅ Smoothness and monotonicity considerations for deep learning models
✅ Applying classical actuarial concepts to deep learning
✅ Credibility transformers and how they enhance deep learning models
✅ An AI vision for the actuarial profession
Time Markers
1:52: Ron’s new insurtech venture and the union between actuarial science and modern technologies.
4:49: Technological shift, commoditization of large language models, and uncertainty quantification.
7:35: Distinguishing between statistics, machine learning, deep learning, and generative AI.
14:00: Are GLMs considered statistical or machine learning models?
17:04: Adoption and deployment trends for machine learning and deep learning models in insurance.
22:14: Neural networks, overparameterization, and regularization techniques.
28:22: Balancing accuracy, complexity, and explainability for deep learning models.
35:00: Addressing smoothness and monotonicity constraints for neural networks, post-job explanations of black box models (ICE).
40:25: Actuarial deep learning and enhancing deep learning models using credibility transformers.
46:54: What the actuarial profession can do to capitalize on the era of neural nets and generative AI.
50:18: Leveraging AI to enhance productivity and improve granularity of reserving analysis.
If you are seeking to explore deep learning and apply it in practice, you want to listen to this.
My Website: maverickactuary.com
The theme for the episode is 𝗗𝗲𝗲𝗽 𝗟𝗲𝗮𝗿𝗻𝗶𝗻𝗴.
Ron and I covered the following topics:
✅ Statistics, machine learning, deep learning, and generative AI
✅ Whether GLMs are statistical or machine learning models
✅ Neural nets and their applicability to insurance
✅ Reducing the risk of overparameterization for neural nets
✅ Balancing accuracy, complexity, and explainability for deep learning models
✅ Smoothness and monotonicity considerations for deep learning models
✅ Applying classical actuarial concepts to deep learning
✅ Credibility transformers and how they enhance deep learning models
✅ An AI vision for the actuarial profession
Time Markers
1:52: Ron’s new insurtech venture and the union between actuarial science and modern technologies.
4:49: Technological shift, commoditization of large language models, and uncertainty quantification.
7:35: Distinguishing between statistics, machine learning, deep learning, and generative AI.
14:00: Are GLMs considered statistical or machine learning models?
17:04: Adoption and deployment trends for machine learning and deep learning models in insurance.
22:14: Neural networks, overparameterization, and regularization techniques.
28:22: Balancing accuracy, complexity, and explainability for deep learning models.
35:00: Addressing smoothness and monotonicity constraints for neural networks, post-job explanations of black box models (ICE).
40:25: Actuarial deep learning and enhancing deep learning models using credibility transformers.
46:54: What the actuarial profession can do to capitalize on the era of neural nets and generative AI.
50:18: Leveraging AI to enhance productivity and improve granularity of reserving analysis.
If you are seeking to explore deep learning and apply it in practice, you want to listen to this.
My Website: maverickactuary.com
D'autres épisodes de "Live With The Maverick"
Ne ratez aucun épisode de “Live With The Maverick” et abonnez-vous gratuitement à ce podcast dans l'application GetPodcast.