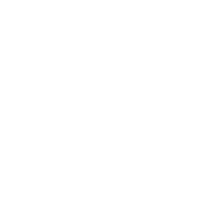
Nail it then Scale it - How to Implement AI in Large Organizations
AI has a lot of potential, but successfully implementing it in large organisations is a tricky business. Everyone is apprehensive and expecting a magic bullet at the same time.
A few dimensions that are crucial to approach AI:
Where do you start, and which projects do you pick?
How do you set up a framework to run AI projects successfully?
Can you navigate all the stakeholders and set the right expectations?
Jonas and Christian dive into these topics in our latest episode of the 10-minute podcast, and walk through practical approaches to embed AI into your company.
#AI #productleadership #productmanagement
--
Partial transcript:
(...)
And generally, it's because we're overcomplicating the use of AI and ML. Through our conversations, we've discussed what is it, really. AI, ML is another tool in your toolbox.
It doesn't change your initial range of use cases that you're looking into as a product leader, but it gives you an extra means to resolve those use cases. So looking to introduce AI and ML in large organizations, it makes sense to split your use cases into two segments. We coin them as working bees use cases and helpers use cases.
So working bees use cases are use cases that are where AI can support by taking over and repetitive user actions, so automating workflows. This with an aim to reduce the time it takes for a user to complete a task, so automation. Helpers are AI solutions where AI is supporting you by providing relevant guidance when you need to make a decision, so decision support.
And looking across and splitting up your set of use cases like that, the working bee use cases, so automation, taking over and repetitive tasks are usually more straightforward to implement and a more clear cut, which in turn will enable you to unlock value faster than helper use cases. So we do recommend looking across your use cases that you start with the bee use cases, so repetitive tasks. Also start simple.
Don't overcomplicate things. Try to start with a simple use case. Try to define a simple solution and get going.
Test and ensure that it works, and then you can work on scaling it across a user base or an application space. Try to de-scope what you want to achieve with AI and ML initially, learn how to use it, see it work, unlock value from it, and then move on from there. Awesome.
I really like your split into two different types of activities, and I have to agree. It's better to start simple, and it's better to start with the easier tasks to be repeated. And so this brings me to another topic, which is in big organizations, one of the effects AI and ML has on people is that there's a certain amount of magic thinking, because you read a lot of stuff in the press.
You read about the, you know, you see amazing pictures being generated, and so there's a lot of magic thinking. So as a product lead, one of the most important things to do in a big organization is to set expectations or you set yourself up to fail. Setting the expectations means you have to dispel some of the magic bullet thinking.
You have to assess the risk of your activities. And by that, I mean, if you do blue sky model training, for example, on certain outcomes on this one, so can you do just blue model thinking, blue model training, blue sky model training? Probably not, because if you do just that, you can fail in every single one of them. You could, mathematically speaking.
If you have advanced analytics, for example, as a avenue for some of the projects you're doing, it's much better, because you can predict the results of some of these projects. So you can have a balanced portfolio. And this is something I've done in the past to explain to the board and to the executive to say, look, I have 50% of projects that are very risky, 50% of products that are more predictable.
(...)
More episodes from "The 10-Minute Product Podcast"
Don't miss an episode of “The 10-Minute Product Podcast” and subscribe to it in the GetPodcast app.