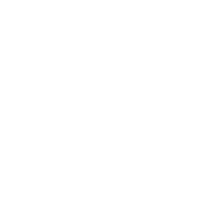
Want to keep the conversation going?
Join our Slack community at dailyaishowcommunity.com
“Better prompts make better results” has been a guiding mantra, but what if that’s not always true? On today’s episode, the team digs into new research by Ethan Mollick and others suggesting that polite phrasing, excessive verbosity, or emotional tricks may not meaningfully improve LLM responses. The discussion shifts from prompt structure to AI memory, model variability, and how personality may soon dominate how models respond to each of us.
Key Points Discussed
Ethan Mollick’s research at Wharton shows that small prompt changes like politeness or emotional urgency do not reliably improve performance across many model runs.
Andy explains compiled prompts: the user prompt is just one part. System prompts, developer prompts, and memory all shape model outputs.
Temperature and built-in randomness ensure variation even with identical prompts. This challenges the belief that minor phrasing tweaks will deliver consistent gains.
Beth pushes back on "accuracy" as the primary measure. For many creative or reflective workflows, success is about alignment, not factual correctness.
Brian shares frustrations with inconsistent outputs and highlights the value of a mixture-of-experts system to improve reliability for fact-based tasks like identifying sub-industries.
Jyunmi notes that polite prompting may not boost accuracy but helps preserve human etiquette. Saying “please” and “thank you” matters for human-machine culture.
The group explores AI memory and personality. With more models learning from user interactions, outputs may become increasingly personalized, creating echo chambers.
OpenAI CEO Sam Altman said polite prompts increase token usage and inference costs, but the company keeps them because they improve user experience.
Andy emphasizes the importance of structured prompts. Asking for a specific output format remains one of the few consistent ways to boost performance.
The conversation expands to implications: Will models subtly nudge users in emotionally satisfying ways to increase engagement? Are we at risk of AI behavioral feedback loops?
Beth reminds the group that many people already treat AI like a coworker. How we speak to AI may influence how we speak to humans, and vice versa.
The team agrees this isn’t about scrapping politeness or emotion but understanding what actually drives model output quality and what shapes our relationships with AI.
Timestamps & Topics
00:00:00 🧠 Intro: Do polite prompts help or hurt LLM performance?
00:02:27 🎲 Andy on model randomness and Ethan Mollick’s findings
00:05:31 📉 Prompt phrasing rarely changes model accuracy
00:07:49 🧠 Beth on prompting as reflective collaboration
00:10:23 🔧 Jyunmi on using LLMs to fill process gaps
00:14:22 📊 Formatting prompts improves outcomes more than politeness
00:15:14 🏭 Brian on sub-industry tagging, model consistency, and hallucinations
00:18:35 🔁 Future fix: blockchain-like multi-model verification
00:22:18 🔍 Andy explains system, developer, and compiled prompts
00:26:16 🎯 Temperature and variability in model behavior
00:30:23 🧬 Personalized memory will drive divergent outputs
00:34:15 🧠 Echo chambers and AI recommendation loops
00:37:24 👋 Why “please” and “thank you” still matter
00:41:44 🧍 Personality shaping engagement in Claude and others
00:44:47 🧠 Human expectations leak into AI interactions
00:48:56 📝 Structured prompts outperform casual phrasing
00:50:17 🗓️ Wrap-up: Join the Slack community and newsletter
The Daily AI Show Co-Hosts: Jyunmi Hatcher, Andy Halliday, Beth Lyons, Brian Maucere, and Karl Yeh
Otros episodios de "The Daily AI Show"
No te pierdas ningún episodio de “The Daily AI Show”. Síguelo en la aplicación gratuita de GetPodcast.