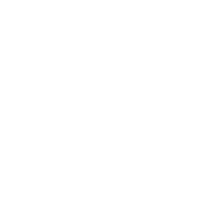
#16 - Alexander Acker - What It Really Takes to Train AI and How to Make It Accessible to Many
How did we get from the first AI models to today's cutting-edge language models, and what’s next for AI infrastructure?
In this episode, I sit down with Alex to explore the history of AI, from early perceptrons to GPT-4, and the often overlooked hardware and system engineering challenges behind modern AI.
We discuss why building and training AI models today is far more than just code and data, it’s about scaling infrastructure, managing distributed GPUs, and creating robust pipelines for fine-tuning and domain-specific AI.
Key highlights include:
- A deep dive into the evolution of neural networks, from perceptrons and backpropagation to transformers and LLMs.
- Why NVIDIA’s early involvement with AI hardware became a game-changer—and how GPUs moved from gaming to powering AI breakthroughs.
- The real cost and complexity behind training large models—and why fine-tuning, LoRA, and model distillation are critical for smaller players in AI.
- A behind-the-scenes look at AI infrastructure challenges: what it takes to train, distribute, and deploy models at scale.
- How Alex’s project Exalsius is building a platform to give AI startups and researchers better, more affordable access to distributed GPU compute, helping democratize AI development.
If you’re curious about where AI is headed, and what it really takes to build models beyond the hype, this is a conversation you don’t want to miss!
Flere episoder fra "Adjmal Sarwary Podcast"
Gå ikke glip af nogen episoder af “Adjmal Sarwary Podcast” - abonnér på podcasten med gratisapp GetPodcast.