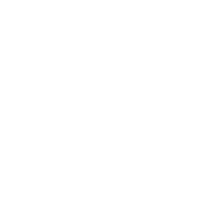
Harvard Research: What if AI Could Redefine Its Understanding with New Contexts?
0:00
6:48
This episode analyzes the research paper titled "In-Context Learning of Representations," authored by Core Francisco Park, Andrew Lee, Ekdeep Singh Lubana, Yongyi Yang, Maya Okawa, Kento Nishi, Martin Wattenberg, and Hidenori Tanaka from Harvard University, NTT Research Inc., and the University of Michigan. The discussion delves into how large language models, specifically Llama3.1-8B, adapt their internal representations of concepts based on new contextual information that differs from their original training data.
The episode explores the methodology introduced by the researchers, notably the "graph tracing" task, which examines the model's ability to predict subsequent nodes in a sequence derived from random walks on a graph. Key findings highlight the model's capacity to reorganize its internal concept structures when exposed to extended contexts, demonstrating emergent behaviors and the interplay between newly provided information and pre-existing semantic relationships. Additionally, the concept of Dirichlet energy minimization is discussed as a mechanism underlying the model's optimization process for aligning internal representations with new contextual patterns. The analysis underscores the implications of these adaptive capabilities for the future development of more flexible and general artificial intelligence systems.
This podcast is created with the assistance of AI, the producers and editors take every effort to ensure each episode is of the highest quality and accuracy.
For more information on content and research relating to this episode please see: https://arxiv.org/pdf/2501.00070
The episode explores the methodology introduced by the researchers, notably the "graph tracing" task, which examines the model's ability to predict subsequent nodes in a sequence derived from random walks on a graph. Key findings highlight the model's capacity to reorganize its internal concept structures when exposed to extended contexts, demonstrating emergent behaviors and the interplay between newly provided information and pre-existing semantic relationships. Additionally, the concept of Dirichlet energy minimization is discussed as a mechanism underlying the model's optimization process for aligning internal representations with new contextual patterns. The analysis underscores the implications of these adaptive capabilities for the future development of more flexible and general artificial intelligence systems.
This podcast is created with the assistance of AI, the producers and editors take every effort to ensure each episode is of the highest quality and accuracy.
For more information on content and research relating to this episode please see: https://arxiv.org/pdf/2501.00070
Flere episoder fra "New Paradigm: AI Research Summaries"
Gå ikke glip af nogen episoder af “New Paradigm: AI Research Summaries” - abonnér på podcasten med gratisapp GetPodcast.