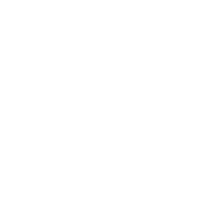
116: Kevin Hu: How data observability and anomaly detection can enhance MOps
What’s up everyone, today we have the pleasure of sitting down with Kevin Hu (Hoo), Co-founder and CEO at Metaplane.
Summary: Dr. Kevin Hu gives us a masterclass on everything data. Data analysis, data storytelling, data quality, data observability and data anomaly detection. We unpack the power of inquisitive data analysis and a hypothesis-driven approach, emphasizing the importance of balancing data perfection with actually doing the work of activating that data. He highlights data observability and anomaly detection as a key to preempting errors, ensuring data integrity for a seamless user experience. Amid the rise of AI in martech, he champions marketing ops' role in safeguarding data quality, making clear that success hinges on our ability to manage data with precision, creativity, and proactive vigilance.
About Kevin
- Kevin did his undergrad in Physics at MIT
- He later collaborated with his biologist sister, assisting in analyzing five years of fish behavior data. This experience inspired him to further his research and earn a master's degree in Data Visualization and Machine Learning
- He also completed a PhD in Philosophy at MIT where he led research on automated data visualization and semantic type detection
- His research was published at several conferences like CHI (pronounced Kai) (human-computer interaction), SIGMOD (database) and KDD (data mining) and featured in the Economist, NYT and Wired
- In 2019, Kevin teamed up with former Hubspot and Appcues engineers to launch Metaplane, initially set out to be a product focused on customer success, designed to analyze company data for churn prevention
- But after going through Y Combinator, the company pivoted slightly to build data analytics-focused tools
- Today Metaplane is a data observability platform powered by ML-based anomaly detection that helps teams prevent and detect data issues — before the CEO pings them about weird revenue numbers.
How to Ask the Right Questions in Data Analysis
When Kevin shared the profound impact César Hidalgo, his mentor at MIT, had on his journey into the data world, it wasn't just about learning to analyze data; it was about asking the right questions. César put together one of our favorite TED talks ever – Why we should automate politicians with AI agents – this was back in 2018, long before ChatGPT was popular.
Hidalgo, recognized not only for AI and ML applications but also developing innovative methods to visualize complex data and making it understandable to a broader audience, was the most important teacher in Kevin’s life. He helped Kevin understand that the bottleneck in data analysis wasn't necessarily a lack of coding skills but a gap in understanding what to ask of the data. This revelation came at a pivotal moment as Kevin navigated his path through grad school, influenced by his sister's work in animal behavior and his own struggles with coding tools like R and MATLAB.
Under Hidalgo's guidance, Kevin was introduced to a broader perspective on data analysis. This wasn't just about running numbers through a program; it was about diffusing those numbers with context and meaning. Hidalgo's approach to mentorship, characterized by personalized attention and encouragement to delve into complex ideas, like those presented in Steven Pinker's "The Blank Slate," opened up a new world of inquiry for Kevin. It was a world where the questions one asked were as critical as the data one analyzed.
This mentorship experience highlights the importance of curiosity and critical thinking in the field of data science. Kevin's reflection on his journey reveals a key insight: mastering coding languages is only one piece of the puzzle. The ability to question, to seek out the stories data tells, and to understand the broader implications of those stories is equally, if not more, important.
Kevin's gratitude towards Hidalgo for his investment in students' growth serves as a reminder of the value of mentorship. It’s a testament to the idea that the best mentors don't just teach you how to execute tasks; they inspire you to see beyond the immediate horizon. They challenge you to think deeply about your work and its impact on the world.
Key takeaway: For marketers delving into data-informed strategies, Kevin's story is a powerful reminder that beyond the technical skills, the ability to ask compelling, insightful questions of your data can dramatically amplify its value. Focus on nurturing a deep, inquisitive approach to understanding consumer behavior and market trends.
Bridging Academic Rigor with Startup Agility
During his career in academia working alongside Olympian-caliber scientists and researchers, Kevin garnered insights that have since influenced his approach to running a startup. The parallels between academia and startups are striking, with both realms embodying a journey of perseverance and unpredictability. This analogy provides a foundational mindset for entrepreneurs who must navigate the uncertain waters of business development with resilience and adaptability.
At the heart of Kevin's philosophy is the adoption of a hypothesis-driven approach. This methodology, borrowed from academic research, emphasizes the importance of formulating hypotheses for various aspects of business operations, particularly in marketing strategies. Identifying the ideal customer profile (ICP), crafting compelling messaging, and selecting the optimal channels are seen not as static decisions but as theories to be rigorously tested and iterated upon. This empirical approach allows for a methodical exploration of what resonates best with the target audience, acknowledging that today's successful strategy may need reevaluation tomorrow.
Another vital lesson from academia that Kevin emphasizes is the respect for past endeavors. In a startup ecosystem often obsessed with innovation, there's a tendency to overlook the lessons learned from previous attempts in similar ventures. By acknowledging and building upon the efforts of predecessors, Kevin advocates for a more informed and grounded approach to innovation. This perspective encourages entrepreneurs to consider the historical context of their ideas and strategies, potentially saving time and resources by learning from past mistakes rather than repeating them.
Key takeaway: Embracing a hypothesis-driven mindset should be familiar grounds for marketers. Challenge your team to identify and test hypotheses around underexplored or seemingly less significant customer segments. This could involve hypothesizing the effectiveness of personalized content for a niche within your broader audience that has been overlooked, measuring engagement against broader campaigns.
Balancing Data Accuracy with Rapid Growth
For startups grappling with survival, the luxury of perfect data is often out of reach. Kevin points out that data quality should be tailored to the specific needs of the business. For instance, data utilized for quarterly board meetings does not necessitate the same level of freshness as data driving daily customer interactions. This pragmatic approach underscores the importance of defining data quality standards based on the frequency and criticality of business decisions.
At the heart of Kevin's argument is the concept that as businesses scale, the stakes of data accuracy and timeliness escalate. He highlights scenarios where real-time data becomes crucial, such as B2B SaaS companies engaging with potential leads or e-commerce platforms optimizing their customer journey. In these cases, even slight inaccuracies or delays can result in missed revenue opportunities or diminished customer trust.
This discourse on data quality transcends the binary choice between perfect data and rapid action. Instead, Kevin advoc...
Flere episoder fra "Humans of Martech"
Gå ikke glip af nogen episoder af “Humans of Martech” - abonnér på podcasten med gratisapp GetPodcast.